OPAC (Online Public Access Catalog)
Pencarian Koleksi UPA Perpustakaan
start it by typing one or more keywords for title, author or subject
Bandwidth selection in kernel density estimation for interval-grouped data
REYES, Miguel - Personal Name
FRANCISCO-FERNANDEZ, Mario - Personal Name
CAO, Ricardo - Personal Name
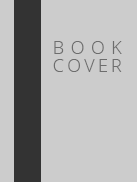
kernel density estimator has to be modified to get a computable and useful approach
in this context. The new nonparametric grouped data estimator needs of the choice
of a smoothing parameter. In this paper, two different bandwidth selectors for this
estimator are analyzed. A plug-in bandwidth selector is proposed and its relative rate
of convergence obtained. Additionally, a bootstrap algorithm to select the bandwidth
in this framework is designed. This method is easy to implement and does not require
Monte Carlo. Both proposals are compared through simulations in different scenarios.
It is observed that when the sample size is medium or large and grouping is not heavy,
both bandwidth selection methods have a similar and good performance. However,
when the sample size is large and under heavy grouping scenarios, the bootstrap
bandwidth selector leads to better results.
EB00000004127K | Available |
Series Title
-
Call Number
-
Publisher
: ,
Collation
-
Language
ISBN/ISSN
-
Classification
NONE
Content Type
E-Jurnal
Media Type
-
Carrier Type
-
Edition
-
Specific Detail Info
-
Statement of Responsibility
Miguel Reyes 1, Mario Francisco-Fernández 2 , Ricardo Cao 2
New collection + Update
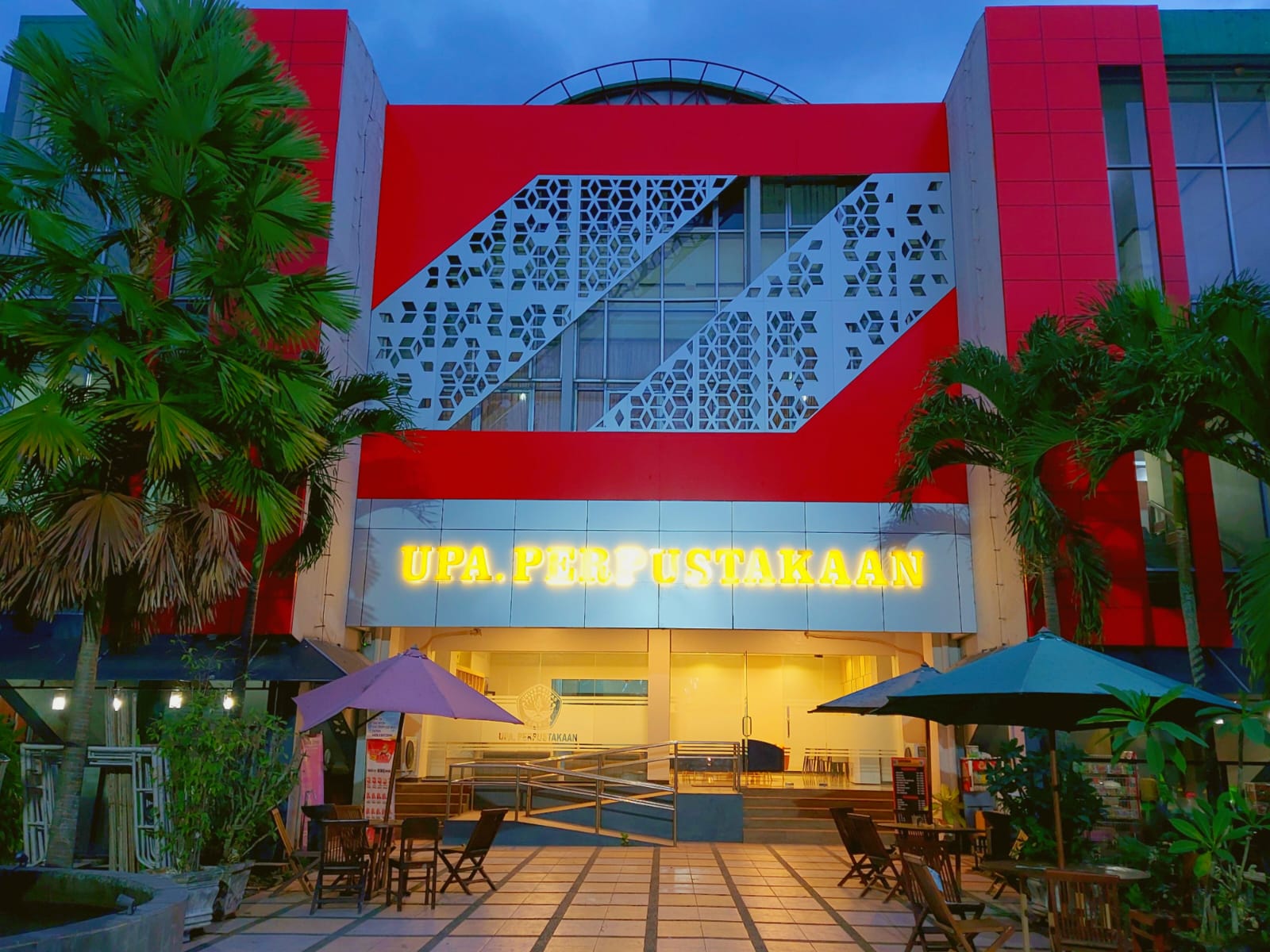
About Us
UPA Perpustakaan
Merupakan Unit Pendukung Akademis (UPA) yang bersama-sama dengan unit lain melaksanakan Tri Dharma Perguruan Tinggi (PT) melalui menghimpun, memilih, mengolah, merawat serta melayankan sumber informasi kepada civitas akademika Universitas Jember khususnya dan masyarakat akademis pada umumnya.
UPA Perpustakaan Universitas Jember sebagai perpustakaan modern dengan layanan prima yang mampu menunjang visi Universitas Jember.
Total Koleksi
Total Item
Total Anggota
Total Pustakawan
Aplikasi Android
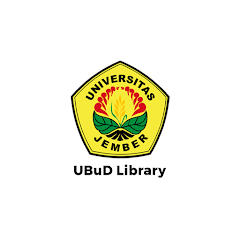
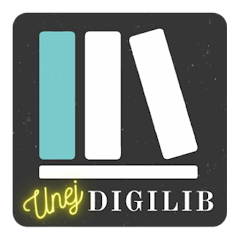
Our Services
Help Desk and Service Support UPA Perpustakaan
Layanan penyerahan Skripsi, Laporan, Thesis, dan Disertasi, Hubungi kami dengan tab/klik untuk mendapatkan informasi lebih lanjut
Layanan Pengembangan dan Kerjasama, Hubungi kami dengan tab/klik untuk mendapatkan informasi lebih lanjut
Layanan peminjaman dan pengembalian koleksi sirkulasi, Hubungi kami dengan tab/klik untuk mendapatkan informasi lebih lanjut
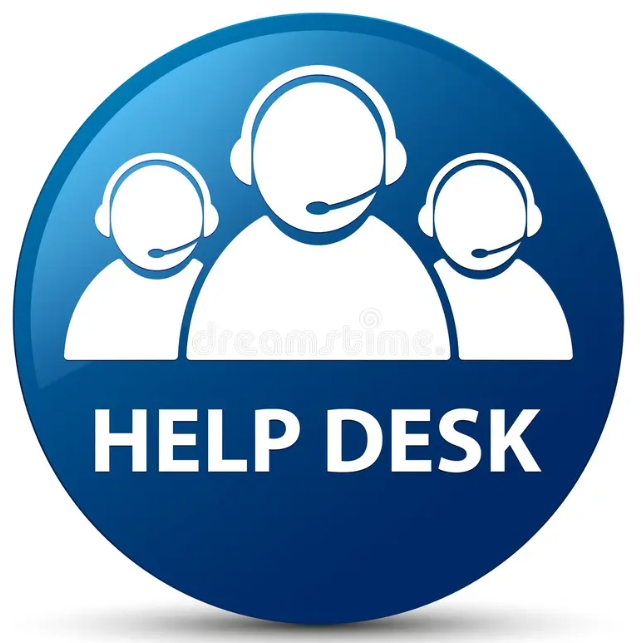
Layanan koleksi Referensi, koleksi Berkala, dan koleksi Skripsi, Hubungi kami dengan tab/klik untuk mendapatkan informasi lebih lanjut
Layanan Karya Deposit Dosen, Hubungi kami dengan tab/klik untuk mendapatkan informasi lebih lanjut
Layanan Registrasi, Hubungi kami dengan tab/klik untuk mendapatkan informasi lebih lanjut