OPAC (Online Public Access Catalog)
Pencarian Koleksi UPA Perpustakaan
start it by typing one or more keywords for title, author or subject
A Nyström spectral clustering algorithm based on probability incremental sampling
Hongjie Jia 1,2 - Personal Name
Shifei Ding 1,2 - Personal Name
Mingjing Du 1,2 - Personal Name
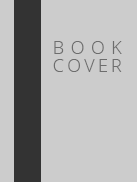
representative sample points to reflect the distribution of data sets much better, this paper designs a dynamic incremental sampling method used for the Nyström spectral clustering, in which the data points are sampled according to different probability distributions and we theoretically prove that the
increase of sampling times can effectively decrease the sam-pling error. The feasibility and effectiveness of the proposed algorithm are analyzed by the experiments on UCI machine learning data sets.
EB00000002615K | Available |
Series Title
-
Call Number
-
Publisher
: ,
Collation
-
Language
ISBN/ISSN
-
Classification
NONE
Content Type
-
Media Type
-
Carrier Type
-
Edition
-
Specific Detail Info
-
Statement of Responsibility
-
New collection + Update
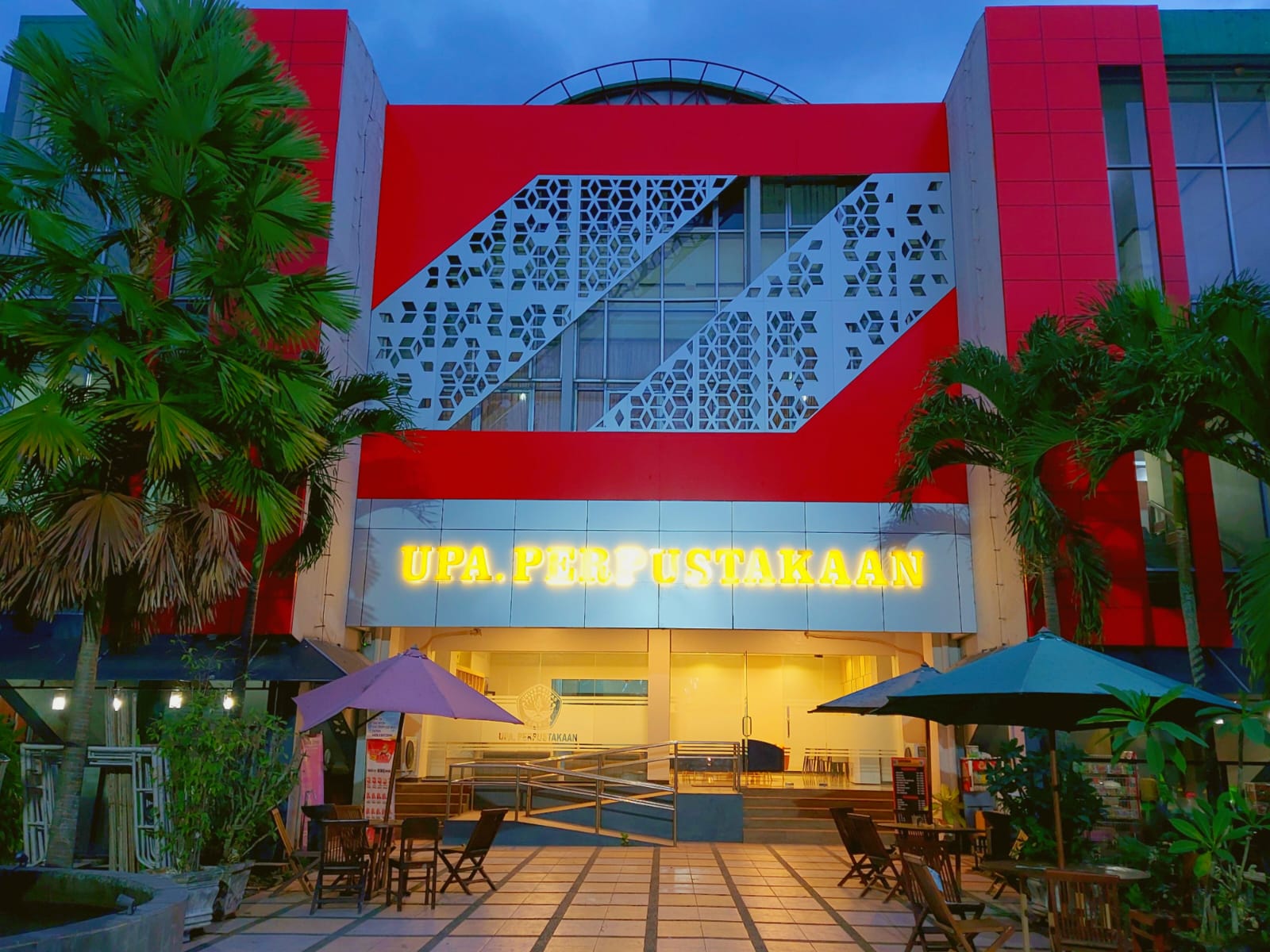
About Us
UPA Perpustakaan
Merupakan Unit Pendukung Akademis (UPA) yang bersama-sama dengan unit lain melaksanakan Tri Dharma Perguruan Tinggi (PT) melalui menghimpun, memilih, mengolah, merawat serta melayankan sumber informasi kepada civitas akademika Universitas Jember khususnya dan masyarakat akademis pada umumnya.
UPA Perpustakaan Universitas Jember sebagai perpustakaan modern dengan layanan prima yang mampu menunjang visi Universitas Jember.
Total Koleksi
Total Item
Total Anggota
Total Pustakawan
Aplikasi Android
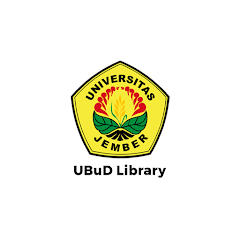
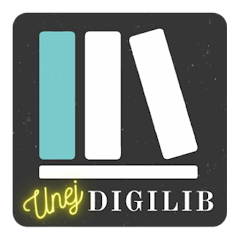
Our Services
Help Desk and Service Support UPA Perpustakaan
Layanan penyerahan Skripsi, Laporan, Thesis, dan Disertasi, Hubungi kami dengan tab/klik untuk mendapatkan informasi lebih lanjut
Layanan Pengembangan dan Kerjasama, Hubungi kami dengan tab/klik untuk mendapatkan informasi lebih lanjut
Layanan peminjaman dan pengembalian koleksi sirkulasi, Hubungi kami dengan tab/klik untuk mendapatkan informasi lebih lanjut
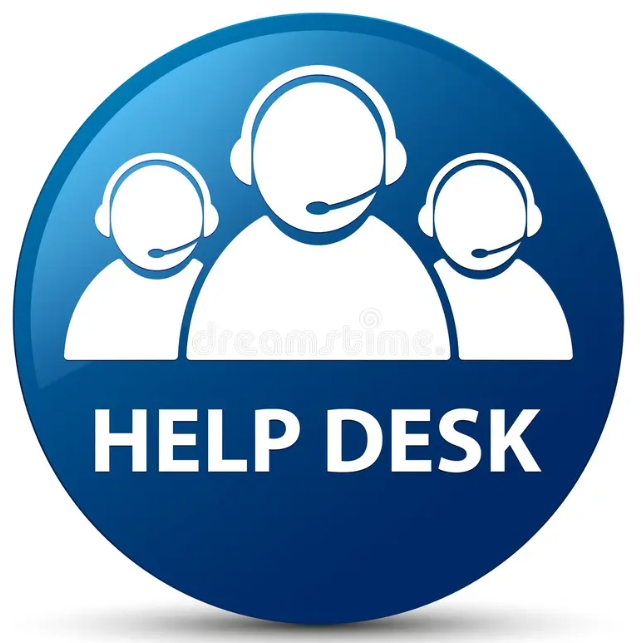
Layanan koleksi Referensi, koleksi Berkala, dan koleksi Skripsi, Hubungi kami dengan tab/klik untuk mendapatkan informasi lebih lanjut
Layanan Karya Deposit Dosen, Hubungi kami dengan tab/klik untuk mendapatkan informasi lebih lanjut
Layanan Registrasi, Hubungi kami dengan tab/klik untuk mendapatkan informasi lebih lanjut